Unraveling the Significance of Signal Transduction Pathways: Key Players in Cancer Development and Progression
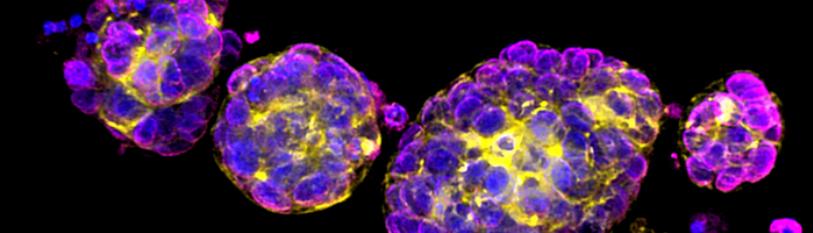
Emad Fadhal*
Department of Mathematics &Statistics, College of Science, King Faisal University, P. O. Box 400, Al-Ahsa 31982, Saudi Arabia
*Corresponding author: Emad Fadhal, Department of Mathematics &Statistics, College of Science, King Faisal University, P. O. Box 400, Al-Ahsa 31982, Saudi Arabia
Citation: Fadhal E. (2023) Unraveling the significance of signal transduction pathways: Key players in cancer development and progression. J Can Ther Res. 3(1):1-9.
Received: July 13, 2023 | Published: August 5, 2023
Copyright© 2023 genesis pub by Fadhal E. CC BY NC-ND 4.0 DEED. This is an open-access article distributed under the terms of the Creative Commons Attribution-NonCommercial-No Derivatives 4.0 International License., This allows others distribute, remix, tweak, and build upon the work, even commercially, as long as they credit the authors for the original creation.
DOI: https://doi.org/10.52793/JCTR.2023.3(1)-28
Abstract
Signal transduction pathways play a central role in cancer development and progression by regulating various cellular processes. Dysregulation of these signaling pathways is common in cancer cells and contributes to their uncontrolled growth and survival. Targeting these aberrant signaling pathways has therefore emerged as a promising strategy to prevent cancer cell proliferation and promote therapeutic outcomes. This study highlights the critical role of signaling pathway activation and regulation in cancer biology. Furthermore, our results identified oncogenes such as PIK3CA, EGFR, AKT1, MAP2K1, AR, JAK2, and JAK1 that are involved in a variety of cellular processes and signaling pathways involved in cell growth, proliferation, survival, metabolism, and signaling. In addition, we identify suppressor proteins such as EP300, TP53, CASP8, PIK3R1, CREBBP, and PTCH1 are of interest in gene regulation, DNA repair, apoptosis, and cell signaling. It is essential to recognize that the roles of these signaling proteins can vary depending on cell type and contextual factors. This study highlights that oncogenes and suppressor proteins critically shape cancer networks and profoundly influence cancer cell behavior. In particular, key proteins identified in different types of cancer, such as TP53, KRAS, CCND1, EGFR, BIRC5, and PIK3CA, underscore their importance in promoting uncontrolled cell growth and proliferation. Continued research on these proteins and their associated signaling pathways will contribute to improved cancer diagnostic and therapeutic strategies.
Keywords
Signal transduction pathways; Neoplasms; Cancer progression; Oncogenes; Tumor suppressor genes
Introduction
Signal transduction pathways play a crucial role in the communication and regulation of cellular processes. These pathways enable cells to respond to external signals such as hormones, growth factors, and nutrients, thereby influencing their behavior and function [1]. Understanding the intricacies of signal transduction pathways is essential for elucidating various biological processes and disease mechanisms. In this context, the disease signal transduction pathway refers to the aberrant functioning or dysregulation of these pathways, leading to the development or progression of certain diseases. Signal transduction pathways are also implicated in immune-related diseases, including autoimmune disorders [2]. Neurodegenerative diseases, such as Alzheimer's disease and Parkinson's disease, are also associated with altered signal transduction pathways [3,4]. Targeting these pathways has shown therapeutic potential in preclinical studies, providing a basis for further investigation into novel treatment strategies for neurodegenerative diseases [5].
Understanding the fundamentals of signal transduction pathways and their dysregulation in disease states is crucial for elucidating disease mechanisms and identifying potential therapeutic targets [6- 7]. One prominent example of a disease closely linked to signal transduction pathways is cancer. The dysregulation of signal transduction pathways is often observed in various types of cancer, playing a pivotal role in tumor genesis and disease progression [8]. The dysregulation of signaling cascades involving pathways such as Ras-MAPK, PI3K-Akt, and Wnt/β-catenin has been implicated in oncogenesis, tumor growth, and metastasis [9-10]. Mutations in the Ras genes or upstream receptors have been found to cause abnormal activation of Ras-MAPK signaling in multiple types of cancer, such as pancreatic, colorectal, and lung cancer. [11-12]. Such discoveries have highlighted the therapeutic potential of targeting these pathways to combat cancer progression [13].
In this study, we leveraged our previously successful analysis of human protein interaction networks to identify key proteins involved in multiple cancer types and their function to improve cancer diagnostic and therapeutic strategies [14,15].
Materials and Methods
In this study, a novel approach was used to treat protein-protein interactions (PPIs) as a metric space and analyze them using graph theory [15]. To achieve this, C++ Boost Graph library (http: //www.boost.org/) was used with an implementation of Dijkstra's algorithm to compute the shortest path between pairs of nodes. The main objective was to identify the network center by identifying nodes with the smallest maximum distance to other nodes and dividing those nodes into zones according to their distance from this central point. This method allowed us to efficiently group the nodes based on their proximity to the network center.
Analysis of pathways and functional enrichment
In this study, a novel approach was used to treat protein-protein interactions (PPIs) as a metric space and analyze them using graph theory [15]. To achieve this, C++ Boost Graph library (http: //www.boost.org/) was used with an implementation of Dijkstra's algorithm to compute the shortest path between pairs of nodes. The main objective was to identify the network center by identifying nodes with the smallest maximum distance to other nodes and dividing those nodes into zones according to their distance from this central point. This method allowed us to efficiently group the nodes based on their proximity to the network center.
Evaluation of signaling pathways associated with oncogenes and tumor suppressor proteins
Evaluations were performed to assess the protein expression levels of oncogenes and tumor suppressors. In this analysis, we used data from extensive cancer genome sequencing studies to identify abundant signaling pathways. Analyzes focused primarily on examining high-scoring interactions, revealing recurring patterns. These interactions often involved genes causally associated with cancer [16].
Proteins essential for cellular processes, signaling, growth, cell cycle regulation, and potential therapeutic targets
In order to evaluate zones of the functional protein interaction network in humans, we identified a set of key human proteins by studying the effects of knocking out their corresponding genes in mice [17,18].
Results
Recently, the importance of protein-protein interactions for understanding cellular function and discovering potential therapeutic targets has been widely recognized. In our previous work, we found that interactions between human proteins could be expressed as a metric space. These spaces group proteins according to their proximity to hub proteins into zones that are highly interconnected and important in biological networks. Our study found that the zone closest to the network center contains essential proteins specialized for certain basic functions. Furthermore, we identified proteins located near the network center as potential targets for therapeutic intervention [19-21].
Based on previous analyses, the current study focuses on all zones of the human functional protein interaction network expressed in signaling pathways implicated in cancer. We limited our investigation to 9448 proteins and mapped them to a comparative toxic genomics database to associate signaling pathway diseases in neoplasms such as breast, prostate, stomach, colon, lung, colon, ovary, and urinary tract. Identified their involvement in various signaling pathways. Tumors of the bladder, liver, and pancreas [22]. Table 1 provides an overview of the identified signaling pathways in neoplasia.
Type of cancer neoplasm |
Number of proteins |
Breast |
149 |
Prostatic |
145 |
Stomach |
79 |
Colorectal |
73 |
Lung |
73 |
Colonic |
47 |
Ovarian |
41 |
Urinary bladder |
40 |
Liver |
39 |
Pancreatic |
38 |
Activation and regulation of signaling pathways play an important role in cancer development and progression. Cancer cells often have deregulated signaling pathways that promote their proliferation and survival. It is therefore important to target these signaling pathways to impair their viability [23-26].
As shown in Table 2, signaling proteins show significant predominance in different forms of signaling pathways in neoplasia, accounting for 28% of the total. It is followed by essential proteins with a 21% share. The remaining functions are distributed as follows: Positive signal (11.7%), cell cycle (11.1%), MAPK cascade (9.3%), negative signal (8.7%), MAPK cascade (36%), and growth (6%).
Cancer neoplasm diseases pathway |
E |
S |
G |
C |
M |
P/S |
N/S |
Breast |
26 (17.4%) |
32 (21.4%) |
5 (10.7%) |
9 (6%) |
9 (6%) |
12 (8%) |
10 (6.7%) |
Prostatic |
24 (16.5%) |
35(24.1%) |
8 (5.5%) |
11 (2.5%) |
11 (2.5%) |
15 (10.3%) |
8 (5.5%) |
Stomach |
18 (22.7%) |
24 (30.3%) |
3 (3.7%) |
10 (12.6%) |
7 (8.8%) |
8 (10.1%) |
8 (10.1%) |
Colorectal |
13 (17.8%) |
15 (20.5%) |
4 (5.4%) |
7 (9.5%) |
7 (9.5%) |
8 (10.9%) |
6 (8.2%) |
Lung |
18 (24.6%) |
25 (34.2%) |
7 (9.5%) |
9 (12.3%) |
11 (15%) |
11 (15%) |
6(8.2%) |
Colonic |
11 (23.4%) |
15 (31.9%) |
2 (4.2%) |
7 (16.7%) |
5 (14.8%) |
8 (17%) |
6 (12.7%) |
Ovarian |
10 (24.3%) |
11(26.8%) |
3 (7.3%) |
6 (14.6%) |
4 (9.7%) |
3 (7.3%) |
3 (7.3%) |
Urinary bladder |
13 (32.5%) |
19 (47.5%) |
5 (12.5%) |
9 (22.5%) |
4 (10%) |
8 (20%) |
7 (17.5%) |
Liver |
9 (23%) |
14 (36%) |
4 (10.2%) |
7 (17.9%) |
6 (15.3%) |
6 (15.3%) |
3 (7.6%) |
Pancreatic |
11 (29%) |
11 (29%) |
2 (5.2%) |
6 (15.7%) |
4 (10.5%) |
6 (15.7%) |
6 (15.7%) |
Average |
21.10% |
28% |
6% |
11.10% |
9.30% |
11.70% |
8.70% |
Cancer neoplasm diseases pathway |
A |
P/A |
N/A |
O |
S |
T |
Breast |
3 (2 %) |
0 (0.0 %) |
1 (0.6%) |
4 (2.6 %) |
3 (2 %) |
6 (4.1%) |
Prostatic |
0 (0.0%) |
0 (0.0%) |
0 (0.0%) |
8 (5.5%) |
3 (2%) |
5 (8.9%) |
Stomach |
2 (2.5 %) |
0 (0.0 %) |
0 (0.0%) |
2 (2.5 %) |
2 (2.5%) |
5 (6.3%) |
Colorectal |
4 (5.4 %) |
1 (1.3 %) |
1 (1.3%) |
4 (5.4 %) |
3 (4.1%) |
4 (5.4 %) |
Lung |
2 (2.7%) |
0 (0.0%) |
0 (0.0%) |
4 (5.4%) |
2 (2.7%) |
4 (5.4%) |
Colonic |
2 (4.2%) |
0 (0.0%) |
1 (2.1%) |
1 (2.1%) |
1 (2.1%) |
2 (4.2%) |
Ovarian |
0 (4.2%) |
0 (0.0%) |
0 (0.0%) |
3(7.3%) |
1 (2.4%) |
2 (4.8%) |
Urinary bladder |
2 (5%) |
0 (0.0%) |
1 (2.5%) |
3 (7.5%) |
3 (7.5%) |
3 (7.5%) |
Liver |
1 (2.5%) |
0 (0.0%) |
0 (0.0%) |
0 (0.0%) |
1 (2.5%) |
3 (7.6%) |
Pancreatic |
1 (2.6%) |
0 (0.0%) |
0 (0.0%) |
1 (2.6%) |
2 (5.2%) |
3 (7.8%) |
Average |
2.40% |
0.10% |
0.50% |
4.1 |
2.90% |
5.10% |
Explore the shared functions of various proteins within the signal transduction diseases pathway involved in cancers neoplasm
We identify TP53 and KRAS proteins that are present in the all cancer neoplasm diseases pathway we are studied. Both TP53 and KRAS have been extensively studied due to their significant roles in cancer development and progression. Understanding their functions and dys-regulation is important for the development of diagnostic tools, targeted therapies, and potential treatment strategies for cancers associated with these genes [35-38]. Moreover, in our study, CCND1, EGFR, BIRC5 and PIK3CA have been observed in various types of cancer and found in many cancer neoplasm signaling pathways associated with uncontrolled cell growth and proliferation [39-41].
Discussion
Our study highlights the critical role of activation and regulation of signaling pathways in cancer development and progression. Cancer cells often have dysregulated signaling pathways that promote their growth and survival. Therefore, targeting these signaling pathways is critical to prevent cancer cell survival and proliferation. The prevalence of signaling proteins in neoplasia, accounting for 28% of the total, highlights their significance in cancer biology. Various functions within signaling pathways, including positive signals, cell cycle regulation, MAPK cascade, negative signals, and growth control, contribute to the intricate network of cellular processes involved in cancer.
Additionally, oncogenes and suppressor proteins play critical roles in cancer networks, and their interactions significantly influence cancer cell behavior. Our study identifies several extensively studied oncogenes such as PIK3CA, EGFR, AKT1, MAP2K1, AR, JAK2, and JAK1, which participate in diverse cellular processes and signaling pathways associated with cell growth, proliferation, survival, metabolism, and signal transduction. We also identify suppressor proteins, including EP300, TP53, CASP8, PIK3R1, CREBBP, and PTCH1, with varying functions related to gene regulation, DNA repair, apoptosis, and cell signaling. However, it is crucial to note that their roles can differ based on the specific cell type and context.
Finally, TP53, KRAS, CCND1, EGFR, BIRC5, and PIK3CAare among the important proteins identified in various types of cancer, underscoring their significance in driving uncontrolled cell growth and proliferation. Further research on these proteins and their associated signaling pathways will contribute to the development of improved diagnostic and therapeutic strategies for cancer.
Conclusions
Our study highlights the pivotal importance of signaling proteins and their functions in cancer development and progression. Oncogenes and suppressor proteins play important roles in cancer networks, and their interactions have profound effects on cancer cell behavior. This study identifies several well-studied oncogenes involved in various cellular processes and signaling pathways, including PIK3CA, EGFR, AKT1, MAP2K1, AR, JAK2, and JAK1. Furthermore, we identify suppressor proteins such as EP300, TP53, CASP8, PIK3R1, CREBBP, and PTCH1 with distinct functions related to gene regulation, DNA repair, apoptosis, and cell signaling. In addition, key proteins identified in different types of cancer, such as TP53, KRAS, CCND1, EGFR, BIRC5, and PIK3CA, underscore their importance in promoting uncontrolled cell growth and proliferation. Further research on these proteins and associated signaling pathways will contribute to improved diagnostic and therapeutic strategies in the fight against cancer.
References
- Sui H, Fan ZZ, Li Q. (2012) Signal transduction pathways and transcriptional mechanisms of ABCB1/Pgp-mediated multiple drug resistance in human cancer cells. J Int Med Res. 40(2):426-35.
- Firestein GS. (2003) Evolving concepts of rheumatoid arthritis. Nature. 423(6937):356-61.
- Hanger DP, Anderton BH, Noble W. (2009) The phosphorylation: the therapeutic challenge for neurodegenerative disease. Trends mol Med. 15(3):112-9.
- Gaki GS, Papavassiliou AG. (2014) Oxidative stress-induced signaling pathways implicated in the pathogenesis of Parkinson’s disease. Neuromolecular Med. 16(2):217-30.
- Ma J, Li H. (2018) The role of gut microbiota in atherosclerosis and hypertension. Front Pharmacol. 9:1082.
- Roskoski R Jr. (2020) Properties of FDA-approved small molecule protein kinase inhibitors: A 2020 update. Pharmacol Res. 165:105463.
- Roskoski Jr R. (2021) Orally effective FDA-approved protein kinase targeted covalent inhibitors (TCIs). Pharmacol Res. 165:105422.
- Hanahan D, Weinberg RA. (2011) Hallmarks of cancer: the next generation. Cell. 144(5):646-74.
- Dhillon AS, Hagan S, Rath O, Kolch W. (2007) MAP kinase signalling pathways in cancer. Oncogene. 26(22):3279-90.
- Polakis P. (2012) Wnt signaling in cancer. Cold Spring Harb Perspect Biol. 4(5):a008052.
- Malumbres M, Barbacid M. (2003) RAS oncogenes: the first 30 years. Nat Rev Cancer. 3(6):459-65.
- Cairns RA, Harris IS, Mak TW. (2011) Regulation of cancer cell metabolism. Nat Rev Cancer. 11(2):85-95.
- Sharma SV, Bell DW, Settleman J, Haber DA. (2007) Epidermal growth factor receptor mutations in lung cancer. Nat Rev Cancer. 7(3):169-81.
- Fadhal E (2023) Exploring the significance of zone 1 in human protein interaction networks: Identifying potential therapeutic targets for cancer treatment. Oncol Radiother. 17(8): 249-53
- Fadhal E, Gamieldien J, Mwambene EC. (2014) Protein interaction networks as metric spaces: a novel perspective on distribution of hubs. BMC Syst Biol. 8(1):1-1.
- Vogelstein B, Papadopoulos N, Velculescu VE, Zhou S, Diaz Jr LA, et al. (2013) Cancer genome landscapes. science. 339(6127):1546-58.
- Wu G, Feng X, Stein L. (2010) A human functional protein interaction network and its application to cancer data analysis. Genome Biol. 11(5):1-23
- Bult CJ, Eppig JT, Blake JA, Kadin JA, Richardson JE. (2012) Mouse Genome Database Group. The mouse genome database: genotypes, phenotypes, and models of human disease. Nucleic Acids Research. 41(D1):D885-91.
- Fadhal E, Mwambene EC, Gamieldien J. (2014) Modelling human protein interaction networks as metric spaces has potential in disease research and drug target discovery. BMC Syst Biol. 8(1):1-2.
- Fadhal E, Gamieldien J, Mwambene EC. (2015) Self-similarity of human protein interaction networks: a novel strategy of distinguishing proteins. Sci Rep. 5(1):1-0.
- Fadhal E. (2023) Exploring the significance of zone 1 in human protein interaction networks: Identifying potential therapeutic targets for cancer treatment. Oncol Radiother. 17 (8):249-53.
- Davis AP, Grondin CJ, Johnson RJ, Sciaky D, McMorran R, et al. (2019) The comparative toxicogenomics database: update 2019. Nucleic Acids Res. 47(D1):D948-54.
- Yang Y, Li X, Wang T, Guo Q, Xi T, et al. (2020) Emerging agents that target signaling pathways in cancer stem cells. J Hemat Oncol. 13:1-8.
- Nouri Z, Fakhri S, Nouri K, Wallace CE, Farzaei MH, et al. (2020) Targeting multiple signaling pathways in cancer: The rutin therapeutic approach. Cancers. 12(8):2276.
- Clara JA, Monge C, Yang Y, Takebe N. (2020) Targeting signalling pathways and the immune microenvironment of cancer stem cells—A clinical update. Nat Rev Clin Oncol. 17(4):204-32.
- He Y, Xu W, Xiao YT, Huang H, Gu D, et al. (2022) Targeting signaling pathways in prostate cancer: Mechanisms and clinical trials. Signal Transduct Target Ther. 7(1):198.
- Levine AJ, Puzio-Kuter AM. (2010) The control of the metabolic switch in cancers by oncogenes and tumor suppressor genes. Science. 330(6009):1340-4.
- Aunoble BÉ, Sanches R, Didier E, Bignon YJ. (2000) Major oncogenes and tumor suppressor genes involved in epithelial ovarian cancer. Int J Oncol. 16(3):567-43.
- Levine RL, Wadleigh M, Cools J, Ebert BL, Wernig G, et al. (2005) Activating mutation in the tyrosine kinase JAK2 in polycythemia vera, essential thrombocythemia, and myeloid metaplasia with myelofibrosis. Cancer cell. 7(4):387-97.
- Visakorpi T, Hyytinen E, Koivisto P, Tanner M, Keinänen R, et al. (1995) In vivo amplification of the androgen receptor gene and progression of human prostate cancer. Nature Gene. 9(4):401-6.
- Davies H, Bignell GR, Cox C, Stephens P, Edkins et al. (2002) Mutations of the BRAF gene in human cancer. Nature. 417(6892):949-54.
- Manning BD, Cantley LC. (2007) AKT/PKB signaling: navigating downstream. Cell. 129(7):1261-74.
- Mendelsohn J, Baselga J. (2000) The EGF receptor family as targets for cancer therapy. Oncogene. 19(56):6550-65.
- Broderick DK, Di C, Parrett TJ, Samuels YR, Cummins JM, et al. (2004) Mutations of PIK3CA in anaplastic oligodendrogliomas, high-grade astrocytomas, and medulloblastomas. Cancer Res. 64(15):5048-50.
- Iyer NG, Özdag H, Caldas C. (2004) p300/CBP and cancer. Oncogene. 23(24):4225-31.
- Harris SL, Levine AJ. (2005) The p53 pathway: positive and negative feedback loops. Oncogene. 24(17):2899-908.
- Chen L, Yang L, Yao L, Kuang XY, Zuo WJ, et al. (2018) Characterization of PIK3CA and PIK3R1 somatic mutations in Chinese breast cancer patients. Nature Commun. 9(1):1357.
- Bell BD, Leverrier S, Weist BM, Newton RH, Arechiga AF, et al. (2008) FADD and caspase-8 control the outcome of autophagic signaling in proliferating T cells. Proc Natl Acad Sci. 105(43):16677-82.
- Bryant KL, Mancias JD, Kimmelman AC, Der CJ. (2014) KRAS: feeding pancreatic cancer proliferation. Trends in Biochem Sci. 39(2):91-100.
- Bartkova J, Lukas J, Müller H, Lützhøt D, Strauss M, et al. (1994) Cyclin D1 protein expression and function in human breast cancer. Int J Cancer. 57(3):353-61.
- Samuels Y, Wang Z, Bardelli A, Silliman N, Ptak J, et al. (2004) High frequency of mutations of the PIK3CA gene in human cancers. Science. 304(5670):554.