The Potential for Data Science Analytics to Remediate Existing Health Disparities Through Improved Clinical and Medical Research Insights
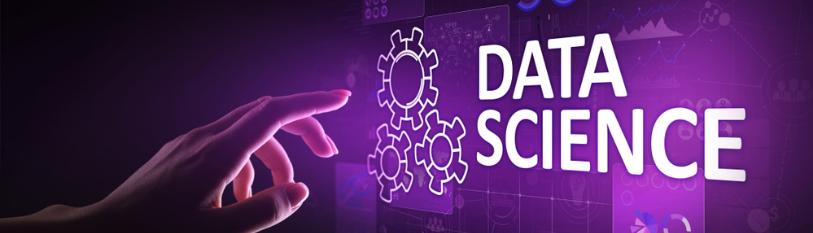
Fatimah Jackson1* and Kayin Davis2
1 Howard University and QuadGrid Data Lab
2 Howard University and QuadGrid Data Lab
*Corresponding author: Fatimah Jackson, Howard University and QuadGrid Data Lab
Citation: Jackson F, Davis K. (2024) The potential for data science analytics to remediate existing health disparities through improved clinical and medical research insights.. Adv Clin Med Res. 5(2):1-10
Received: March 5, 2024 | Published: March 25, 2024
Copyright© 2024 genesis pub by Jackson F, et al. CC BY-NC-ND 4.0 DEED. This is an open-access article distributed under the terms of the Creative Commons Attribution-Non Commercial-No Derivatives 4.0 International License., This allows others distribute, remix, tweak, and build upon the work, even commercially, as long as they credit the authors for the original creation.
Abstract
Data science analytics can respond definitively to health disparities through an investment in the creation of databases that emphasize the unstructured, qualitative nature of the most relevant information needed to alleviate health disparities. This paper discusses the potentials for unstructured machine learning to harness qualitative, ethnographic information of relevance in reconstructing the origins and maintenance of current inequities in diverse populations, medical settings, and health conditions. We also identify the current databases that attempt to address health disparities, and we suggest explicit strategies that must be put in place to use data science analytics to identify the nuanced details of health inequities. The application of ethnographic-rich databases to clinical and medical research can increase their power and better identify the proximate and ultimate causes in understudied health disparities.
Keywords
Machine learning; Artificial intelligence; Understudied populations; Ethnographic data
Introduction and Background
Data science analytics has become an integral part of clinical and medical research. A recent report [1] suggests that the application of data science resources can enhance our ability to reduce health disparities affecting communities across the country. Data science analytics benefits from its interdisciplinary nature. It relies upon insights from a variety of fields in the format of structured and unstructured data. This information is systematically presented and extracted using data mining techniques, machine learning algorithms, and these are the foundation for big data. The healthcare industry generates large quantities of big data which can be harvested to address specific hypotheses in health disparities. Data science analytics provides aid to process, manage, analyze, and assimilate the large quantities of fragmented, structured, and unstructured data created by healthcare systems [2]. In this paper, we consider the potential for data science to help resolve longstanding deficiencies in health underlying health disparities (see 3), particularly when it is culturally contexed, socially informed, and appropriately applied. We build on the perspective that the translation process in health care could be accelerated if representative data were gathered and used in more innovative and efficient ways [4].
The roles of data, science, and healthcare are to provide practical insights and to aid in the strategic decision-making process. The other goal is to provide practical insights and optimization solutions concerning the health system. Data science analytics helps build a comprehensive view of patients, consumers, and clinicians. Data-driven decision-making often reveals new possibilities to boost healthcare quality and quantity [2,5].
Currently, clinical data scientists combine their knowledge of both data science analytics and clinical medicine to transform raw data into meaningful information. They work with electronic health records (EHRs), medical images, genomics data, and other healthcare datasets to uncover hidden patterns, identify risk factors, and develop predictive models to nudge us closer to the gold standard of precision medicine. In the hands of clinically oriented data scientists, data-driven research approaches and ensures subject protection as well as the reliability and credibility of trial results. However, this approach alone may not be enough to significantly remediate existing health disparities. This is because health disparities are not simply the consequence of inadequate analyses. Too often, we also lack the socially informed and culturally contexed raw data to make sophisticated insights and sustainable decisions about healthcare, particularly for marginalized and underrepresented populations and individuals. In this paper we identify and review the current uses of Big Data to address health disparities, consider the structure of data science analytics, and point out where this field can be used more effectively to remediate current deficiencies within health research in understudied ethnic groups.
The Current Status of Health Disparities in the USA
Health disparities in the USA are a significant national concern. Inequities exist across various dimensions such as race, ethnicity, socioeconomic status, and geographic location. Some of the current major health disparities include the linked inequities in mortality rates, life expectancy, the burden of disease, and mental health. For example, the gap in mortality rates between older European-American and African American adults remains, although it has narrowed for individuals in urban areas in the last 60 years. The disparity in mortality is also reflected in the differences in life expectancy between African Americans and European Americans. When we consider the burden of disease carried by various populations, the inequities persist and with them, important differences in the incidence and intensity of mental health disabilities.
Compounding this population variation in health status is the lack of insurance coverage. The absence of insurance coverage significantly impacts the seeking of healthcare which itself is not evenly distributed in the country. These disparities are due to differential exposure to environmental risks, access to health care, and socioeconomic opportunities and resources. Additionally, our databases are often imbalanced and do not represent those in extreme poverty and severe disadvantage. Their poverty is, in fact, a barrier to equity in health.
Given the persistence of health disparities and the increasing capabilities of data science analytics, we hypothesize that the discipline can be tailored to explicitly address and rectify existing health inequities through the systematic application of unstructured machine learning and the use of AI to transform unstructured data into structured data.
Health Disparities Databases
There are several databases that provide valuable data on health disparities. These include the following—
-
National Healthcare Quality and Disparities Reports from the Agency for Healthcare Research and Quality (AHRQ) reports on healthcare quality and disparities.
https://www.ahrq.gov/research/findings/nhqrdr/index.html
-
2022 National Healthcare Quality and Disparities Report Data Sources from AHRQ provides data sources for the National Healthcare Quality and Disparities Report. 2022 National Healthcare Quality and Disparities Report - NCBI Bookshelf (nih.gov)
-
Data at WHO - World Health Organization (WHO) The WHO Health Inequality Monitor provides evidence on existing health inequalities and makes available tools and resources for health equity monitoring. https://www.who.int/data
-
Using Data to Reduce Disparities and Improve Quality – CHCS from the Center for Health Care Strategies (CHCS) discusses how national datasets such as U.S. Census, Behavioral Risk Factor Surveillance System (BRFSS), or RWFJ’s Country Health Rankings can provide context to national and state trends and identify some of the most salient social determinant of health data. https://www.chcs.org/resource/using-data-to-reduce-disparities-and-improve-quality-a-guide-for-health-care-organizations/
-
MEDLINE®/PubMed® Search and Health Disparities & Minority Health from the National Library of Medicine provides information resources on health disparities and health in ethnic minority populations. https://www.nlm.nih.gov/services/queries/health_disparities_details.html
-
Health Disparities Report 2021 - America’s Health Rankings uses publicly available data sources like the American Community Survey (ACS), the Centers for Disease Control and Prevention’s (CDC) Behavioral Risk Factor Surveillance System (BRFSS), the Current Population Survey’s Food Security Supplement (CPS-FSS) and the National Vital Statistics System (NVSS). https://www.americashealthrankings.org/learn/reports/2021-disparities-report
-
All of Us Database is a key component of the federally initiated Precision Medicine Initiative (PMI). Precision, medicine is an innovative method that considers individual differences in genetics, lifestyles, and environments to develop personalized care. The PMI is aimed at replacing the “one-size-fits-all” approach to healthcare by bringing together researchers, health care providers, and patients to work together and is an excellent new approach to expand the database to reduce health disparities. https://www.pcrm.org/news/good-science-digest/national-institutes-healths-all-us-research-project-launches-promises?gad_source=5&gclid=EAIaIQobChMI5Y3PpMrJhAMVQkdHAR0w9AaREAAYAiAAEgJsgfD_BwE
These databases provide valuable insights into health disparities and suggest strategies for reducing them, however all except the All of Us database appear largely exogenous to the understudied populations in need of representation. Except for the All of Us database, these databases are not explicitly designed to address understudied groups and the specific issues of these groups that put them at risk for health disparities. We posit that the most important issues underlying health disparities are often below the surface, ethnographically qualitative variables that require expanded databases specifically constructed to identify, harvest, and analyze these kinds of information.
Key Methods in Data Science Analytics
Data science analytics relies upon a set of specific techniques to identify, gather, ingest, store and process, analyze, and communicate data. The field is broadly encompassing and includes everything from simply analyzing data to theorizing ways of collecting data and creating the frameworks needed to store it. Within clinical and medical research applications of data science analytics, key methods include machine learning and artificial intelligence. More broadly, data science analytics is a multidisciplinary field that uses a combination of math, statistics, specialized programming, advanced analytics, artificial intelligence (AI), and machine learning to uncover actionable insights hidden in an organization’s data base. These insights can guide decision-making and strategic planning in medical and clinical research, but before they can be applied, relevant data needs to be collected. Machine learning is the tool most often used.
In the context of machine learning, data can be broadly classified into two types: structured and unstructured. The main steps remain the same: researchers collect training data, they use this to train a model, they then can evaluate it and eventually deploy it in model development and implementation. However, depending upon whether a researcher uses structured or unstructured data, the results can look somewhat distinct. Converting unstructured data, which is more difficult to quantify, into semi-structured or structured data is time-consuming, but makes it more actionable for addressing health disparities. AI, discussed below, is often used in making unstructured data more quantifiable.
Basically, structured data is organized, follows a specific blueprint or format, fits neatly into the rows and columns of a database, and is easy to analyze. It is more predictable and within the context of health disparities research, it includes data such as zip codes, environmental toxicity scores, regional disease indices, and other data that can be easily searched using SQL. Structured data shines in situations requiring quantitative, data-driven insights, but to adequately address health disparities, we often must dig deeper into the salient social, cultural, and economic patterns that contribute to health inequities.
However, the richest sources of health disparity-remediating data are often in unstructured formats. It is generally more difficult to categorize or search unstructured formats and these include sources such as photos, videos, podcasts, social media posts, podcasts, and emails. Most of the data in the world is unstructured and qualitative (rather than quantitative) in nature. It has no specific format, making it more difficult to systematically document. Unstructured data is the preferred province of the qualitative social sciences, and indeed from these disciplines researchers can develop reproducible techniques to make this unstructured data better fit into standard data science analytics database formats, making it easier for computers to understand and analyze. Within the context of health disparities research, some examples of sources of unstructured data include-
-
Emails: Emails contain important information in a variety of forms, including text, images, and maybe even video and audio files containing lyrics of songs, speeches of social influencers, information about traditional medicines.
-
Text files: Word processing, spreadsheets, PDF files, reports, and presentations are additional sources of unstructured data that can provide insights into the context of health disparities. For example, a group’s vernacular may be expressed in text files that contain hidden insights into medical priorities and taxonomies.
-
Websites: Websites such as YouTube, photo sharing sites, Instagram, are excellent unfiltered sources of unstructured information from underrepresented populations.
-
Social media: Data generated from social media platforms such as Facebook, X, and Linkedin can have a huge impact on clinical and medical research since so much clinical and medical information is exchanged through these platforms. Additionally, increasing numbers of people use social media networks as a primary means of socializing, so it can provide important insights into how understudied groups interact, what is valued, and what are their assumptions about health and wellness.
-
Multimedia files: Images, videos, and audio files may contain information on a targeted group’s religious and spiritual practices influencing health, codified body language, dance moves that express values and priorities affecting health care, and other qualitative sources of insights into targeted groups.
Analyzing and applying these types of information is important to developing a comprehensive profile of underrepresented communities in health disparities research. This gives a framework for contextualizing qualitative cultural information for reformatting into unstructured machine learning. Developing a comprehensive profile is also vital since many of the key elements affecting health are deeply embedded in non-traditional sources of cultural, social, and economic information. Unstructured machine language can identify and ultimately analyze the more fluid aspects of the cultures of vulnerable groups. Evaluating and applying these types of information is foundational if researchers want to successfully manage data quality metrics and usability [6] and generate sustainable interventions for current health disparities. We need databases that make sense from endogenous, emic perspectives but are translatable for general consumption.
In general, then the data science analytics lifecycle involves various roles, tools, and processes, which enable analysts to glean actionable insights. Typically, a data science analytics project to sequester information to alleviate health disparities begins with data collection from all relevant sources using a variety of methods. As previously suggested, the most salient of these sources for new insights into health disparities will be from unstructured data, much of which can be accessed ethnographically. Once these data are collected, they must be cleaned, deduplicated, transformed, and combined using extraction, transformation, and loading (ETL) jobs and other data integration techniques and technologies. Data analysis begins with researchers conducting a set of exploratory data analyses to examine for biases, patterns, ranges, and to determine the distributions of values within the data. Once the analyses are confirmed, the resulting insights are communicated as reports and other data visualizations such that non-specialists can understand and apply the results. Data science analytics is already one of the fastest-growing fields in clinical and medical research. The addition and intentional use of unstructured machine learning data on the qualitative contributors to health disparities will only enhance its utility and provide the best option to remediate these health inequities.
An important new tool in data science analytics is artificial intelligence or AI. This technological innovation represents the intelligence of machines or more accurately, the software programming of machines as opposed to the intelligence of organic organisms. AI comes out of the discipline of computer science as it develops and improves upon the intelligent capabilities of machines. AI technology is extensively used in government, industry, and science and the latest incarnations involve building machines capable of learning, using reason, and acting in a way that highly imitates human intelligence and, in some cases, exceeds human intelligence.
The major problem with initiating artificial intelligence is to efficiently divide it into sub problems. These are the specific capabilities that are expected in an intelligent system. AI draws upon the social and life sciences and the integration of these data has begun to improve the intellectual breadth and capabilities of AI and enhance their capabilities to remediate health disparities. To date, Intelligent machines have yet to completely replicate the capabilities of human intelligence.
Artificial Intelligence (AI) can be classified in several ways. Artificial Narrow Intelligence (ANI), also known as Weak AI, is designed to perform a single or narrow task, often faster and better than a human mind can. However, it can’t perform outside of its defined task. Examples of ANI include Siri, Amazon’s Alexa, IBM Watson, and OpenAI’s ChatGPT. These rely on reactive and limited memory machines. On the other hand, Artificial General Intelligence (AGI) is a theoretical concept at present, can use previous learnings and skills to accomplish new tasks in a different context without the need for human beings to train the underlying models. This is an expansion of a previously limited memory machine since this system can learn from historical data fed into its program and therefore has a broader context for reasoned interpretation and action. This ability allows AGI to learn and perform any intellectual task that a human being can. For AI to truly discern the origins and persistence of health disparities in depth, we will need a theory of mind type of AI. This type of AI can understand others’ beliefs, intentions, desires and incorporate this information into intervention projections. The final improvement is Artificial Superintelligence. This is a hypothetical AI that surpasses human intelligence and capability. It is self-aware, has its own consciousness, and recognizes its own state. We are not yet at this level computationally. No existing programs have attained this level of competence and indeed, it will be unlikely without the inclusion of a broader resource base to train AI.
Health disparities in need of interventions from data science analytics
Health disparities in the USA are a significant concern. They exist across various dimensions such as race, ethnicity, socioeconomic status, sexual orientation, and geographic location. There are widespread disparities in clinical and medical specialties including neonatology, emergency medicine, surgery, psychiatry, and anesthesiology. Racial disparities exist in emergency medical services, and significant racial/ethnic differences have been observed in obesity, a harbinger of many other adverse medical issues.
Databases that provide comprehensive overview of health disparities and inequalities across a wide range of diseases and disorders include the CDC Health Disparities and Inequalities Report and Healthy People 2020. These databases reveal that current disparities are due to differential exposure to environmental risks, access to health care, and socioeconomic opportunities and resources. However, the goal of identifying the overt and covert barriers to health equity remains incomplete. Data science analytics can play a crucial role in reducing health disparities by leveraging data to identify, investigate, and intervene in health inequities. There are some specific ways that the discipline can be tailored to reduce health disparities.
Data science analytics can help identify disparities in health outcomes by analyzing data on race, ethnicity, gender, age, and socioeconomic factors using a variety of models, not just the stereotypic formulations. This can help researchers understand where disparities exist and who is most affected since multiple and diverse analyses may find new correlations for investigation. Once disparities are identified, data science analytics can be used to investigate the root causes of these disparities. This could involve analyzing social determinants of health, such as income, education, and living conditions, but also delving into the unstructured aspects such as spiritual beliefs, use of traditional therapies, historical interactions with the clinical and medical establishment, etc. Data science analytics can also help craft targeted interventions to reduce health disparities. This could involve developing predictive models to identify individuals at risk or using data to evaluate the effectiveness of interventions. This would need to be done at a local level since the relevant variables differ geospatially and socioeconomically. Data science analytics can help improve access to quality care by identifying areas where access to optimal health care is limited and suggests ways to improve. Data science analytics can help researchers better understand the social determinants of health and how they contribute to health disparities. This could involve analyzing data on factors like poverty, education, and housing and the impacts of inequalities in these areas on individual and group susceptibilities to disease and disorder. Finally, by bringing health care disparities into the spotlight, data science analytics can help promote health equity and effect real change in vulnerable groups. While data science analytics is just one tool, it can be a very powerful one with expanded databases and intentional attention to the issues of most relevance for disenfranchised populations.
Lee and Viswanath identified the two main problems with Big Data in the context of health disparities-- data absenteeism (lack of representation from underprivileged groups) and data chauvinism (faith in the size of data without considerations for quality and contexts) [7]. With increased sample sizes, the application of higher-level analytics, and more integrative analysis we can expect decreased health disparities and increased participation of marginalized groups in healthcare. Recently published articles on data science analytics in clinical and medical research have already begun to identify gaps in our databases in response to specific areas of health. For example, improving the quality of epidemiologic data are expected to accelerate improvements in child health outcomes [8], Big Data has been used to identify risk for caries and its correlations with diverse social determinants of health [9], and data science analytics approaches have been used to predict childhood cognitive patterns. AI has been tailored to predict diabetes severity [10] and a call has been made to revise AI applications in retinal disease so that they show less inaccuracy in minority populations [11]. We seem to be well on our way to broadening the evaluative ability of AI through improvements in the core programming so that it is more inclusive. Indeed, this seems to be the direction recommended by other data science experts who have recently published on this issue [12, 13].
It is important to emphasize that attaining the anticipated impact will require concerted and directed efforts in data science analytics. Researchers must increase the breadth and depth of databases included in our analyses, increase the incorporation of evolutionary perspectives into database research design (e.g., niche construction theory) and judiciously follow these six essential steps of decomposition, pattern recognition and modeling, abstraction, algorithmic design and automation, assessment, and analysis and finally documentation.
Daunting health disparity problems need to be broken down into smaller and manageable parts by identifying the genomic and ethnohistoric information geospatially to intentionally include underrepresented groups. Ethnographic techniques have been used to successfully identify nuance in the health disparities experienced by autistic populations, epigenomics, homosexual Black and Latino men, within hospital intensive care units, among Native Americans, and in breastfeeding situations [14 -20]. Researchers can use the qualitative data formatted for unstructured machine learning to identify similarities and regularities among the populations that are most vulnerable to health disparities of interest. They then need to focus on the essential details and ignore the irrelevant ones that often hitchhike with unstructured qualitative data. Researchers can then create a systematic template or flowchart of the steps used to address a specific health disparity in a particular population and use computers to reproducibly perform the tasks efficiently and accurately. Finally, by evaluating the solution and its outcomes, researchers can verify that gaps in the existing databases have been filled with relevant and appropriate data. Of course, the ultimate step for data science analysts is to write up the results to assess the efficacy of this suggested approach as the aim is to develop a scientifically rational and sustainable remediation effort.
Declarations
Ethics approval and consent to participate
All data discussed in this paper are from published databases.
Consent for Publication
All authors of this paper agree to its submission for publication consideration.
Availability of Data and Materials
All databases consulted in this paper are already in the public domain.
Competing Interests
The authors declare no competing interests.
Funding
This work is supported by the Division of Engagement and Outreach, All of Us Research Program, National Institutes of Health under award number 1OT20D028395-01, a small grant from QuadGrid Data Lab, and publication expenses from the Department of Biology, Howard University.
Authors' Contributions
The paper was conceived and written by FJ and extensively reviewed by KD. Both authors participated in the approval of the final version.
Acknowledgements
The authors developed this paper for the Special Issue of Advances in Clinical and Medical Research.
References
-
Huerta J, Senn W, Prybutok G, Prybutok VR. (2023) Addressing Health Disparities in Public Health Through the Application of Data Science Software in the Last 5 Years: A Preferred Reporting Items for Structured Review and Meta-Analyses Structured Review. CIN: Comput Informa Nurs. 41(5):267-74.
-
Subrahmanya SVG, Shetty DK, Patil V, Hameed BMZ, Paul R, et al. (2022) The role of data science in healthcare advancements: applications, benefits, and future prospects. Ir J Med Sci 191(4):1473–83,
-
Dankwa‐Mullan I, Zhang X, Le PT, Riley WT. (2021) Applications of big data science and analytic techniques for health disparities research. The science of health disparities research, 221-242.
-
Breen N, Berrigan D, Jackson JS, Wong DWS, Wood FB, et al. (2019) Translational Health Disparities Research in a Data-Rich World. Health Equity. 3(1):588-600.
-
https://www.institutedata.com/blog/what-is-clinical-data-science-an-overview-of-the-field/.
-
https://www.coursera.org/articles/structured-vs-unstructured-data
-
Lee EW, Viswanath K. (2020) Big data in context: addressing the twin perils of data absenteeism and chauvinism in the context of health disparities research. J Med Internet Res. 22(1):e16377
-
Vesoulis ZA, Husain AN, Cole FS. (2023) Improving child health through Big Data and data science. Pediatr Res. 93(2):342-49.
-
Rodriguez JL, Thakkar-Samtani M, Heaton LJ, Tranby EP, Tiwari T. (2023) Caries risk and social determinants of health: A big data report. J Am Dent Assoc. 154(2):113-21.
-
AlZu’bi S, Elbes M, Mughaid A, Bdair N, Abualigah L, et al. (2023) Diabetes monitoring system in smart health cities based on big data intelligence. Fut Internet, 15(2):85.
-
Jacoba CMP, Celi LA, Lorch AC, Fickweiler W, Sobrin L, et al. (2023) Bias and Non-Diversity of Big Data in Artificial Intelligence: Focus on Retinal Diseases: “Massachusetts Eye and Ear Special Issue”. In Seminars in Ophthalmology. Taylor & Francis. 1-9.
-
Zhang X, Pérez-Stable EJ, Bourne PE, Peprah E, Duru OK, et al. (2017) Big data science: opportunities and challenges to address minority health and health disparities in the 21st century. Eth Dis. 27(2):95.
-
Bowe AK, Lightbody G, Staines A, Murray DM. (2023) Big data, machine learning, and population health: predicting cognitive outcomes in childhood. Pediatr Res. 93(2):300-07.
-
Sinding C. (2010) Using institutional ethnography to understand the production of health care disparities. Qual Health Res. 20(12):1656-63.
-
Lock M, Argentieri MA, Shields AE. (2021) The contribution of ethnography to epigenomics research: toward a new bio-ethnography for addressing health disparities. Epigenomics, 13(21):1771-86.
-
Singh JS, Bunyak G. (2019) Autism disparities: A systematic review and meta-ethnography of qualitative research. Qual Health Res. 29(6):796-08.
-
Cricco-Lizza R. (2007) Ethnography and the generation of trust in breastfeeding disparities research. Appl Nurs Res. 20(4):200-04.
-
Yarahmadi S, Soleimani M, Gholami M, Fakhr-Movahedi A, Madani SMS. (2024) Applying Carspecken's critical ethnography method to uncover the culture of health disparity in intensive care units. Nursing Practice Today, X-X.
-
Brown DS. (2007) The Barrier of Fear: An Ethnographic Interview About Native American Health Disparities. Perm J. 11(1):62-64.
-
Mutchler MG, McKay T, McDavitt B, Gordon KK. (2013) Using peer ethnography to address health disparities among young urban Black and Latino men who have sex with men. Am J Public Health. 103(5):849-52.
This article was originally published in a special issue entitled “Integrating Data Science into Clinical and Medical Research”, handled by Editor Dr. Fatimah Jackson.